How Qlik Cut Escalations by 30% in 6 Months—Without Hiring a Single New Agent
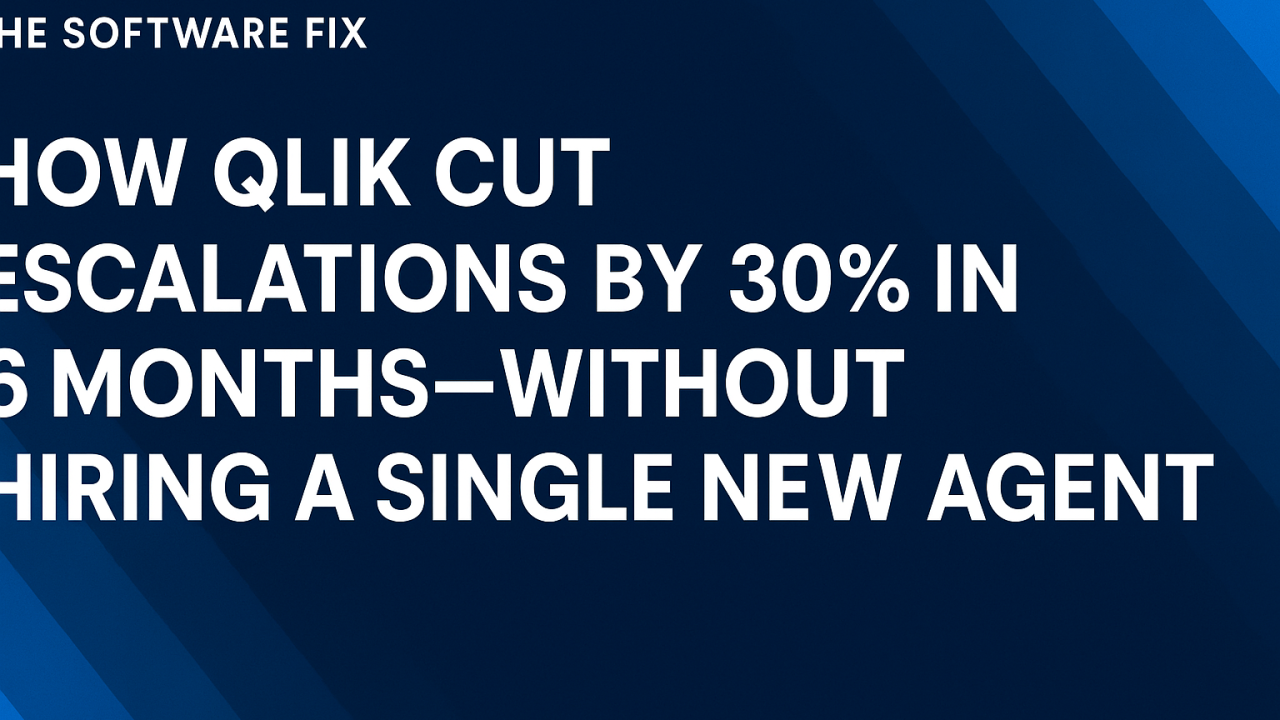
AI, Sentiment Analysis, and a Proactive Mindset Reshape the Modern SaaS Support Team By
Scaling your support team doesn’t have to mean adding more headcount. Qlik, the data analytics platform, has proven just that. Facing a rising volume of support cases and pressure to maintain high customer satisfaction, the company turned to AI and sentiment-driven insights to transform its support operations—ultimately reducing escalations by 30% in just six months.
Their approach offers a repeatable blueprint for SaaS companies looking to maximize the output of their existing teams without compromising on quality or outcomes.
1. The Hidden Cost of Escalations
Support escalations are more than just a customer headache—they’re expensive. Every time a ticket bypasses frontline support and lands in engineering or customer success, resolution time lengthens, morale dips, and cost per case skyrockets.
At Qlik, executives recognized that they needed to reverse this trend. But rather than hire more agents, they focused on smarter case handling.
That meant moving from a reactive support model—where agents waited for something to go wrong—to a proactive one, where they could identify issues earlier and intervene intelligently.
2. From Reactive Surveys to Real-Time Signals
Traditionally, companies have relied on lagging indicators like CSAT scores or post-resolution NPS surveys to evaluate customer experience.
Qlik knew that by the time these metrics were available, it was already too late to save the customer experience.
The company implemented real-time monitoring tools that analyzed both structured ticket data and unstructured text (like customer comments and support threads). AI models then produced dynamic Sentiment Scores and Attention Scores, flagging cases that might be headed for escalation even before the customer complained.
This gave Qlik’s team a predictive edge: they could prioritize tickets not just based on SLA, but on emotional and contextual urgency.
3. The Role of Customer Engineering
Another key lever in Qlik’s transformation was the strategic deployment of customer engineers—technical professionals embedded within the support org who act as both troubleshooters and consultants.
By pairing AI-driven case intelligence with these hybrid roles, Qlik was able to resolve complex issues faster while offering a more human, consultative experience.
Importantly, customer engineers weren’t just responding to problems. They were equipped with dashboards that surfaced high-risk accounts, allowing them to initiate check-ins or provide preventive guidance before issues arose.
4. Smaller Team, Better Performance
Despite maintaining headcount, Qlik saw measurable improvement in performance. Within six months, the company had reduced escalations by 30% for its analytics platform. The support team also reported better morale and less burnout, thanks to clearer priorities and fewer fire drills.
The results challenged the assumption that growth must always be accompanied by scale. Instead, Qlik showed that with the right tooling and data, a lean support team can outperform a larger one.
5. Building a Blueprint for Others
Qlik’s model offers a compelling template for other SaaS organizations:
- Start with sentiment: Implement AI tools that parse customer tone and engagement to generate real-time case health scores.
- Flag before it burns: Set triggers that alert managers or customer engineers before a case deteriorates.
- Layer in expertise: Introduce customer engineering roles that can move quickly on technical or strategic concerns.
- Don’t wait for surveys: Build systems that respond to live data, not end-of-journey surveys.
- Align incentives to prevention: Shift KPIs from average resolution time to avoided escalations and proactive interventions.
This isn’t just theory—it’s working in production today.
Conclusion
Qlik’s support transformation underscores a broader trend in SaaS: smaller, smarter teams powered by AI and strategic human capital. Rather than treating AI as a chatbot gimmick or afterthought, the company embedded it into every layer of support decision-making. Combined with a new generation of customer engineers, this hybrid model delivers not just efficiency—but trust, satisfaction, and long-term loyalty.
For companies seeking to do more with less, the future of support might already be here.
Sources:
- Qlik Case Study – SupportLogic
- Interview insights and product overviews from SupportLogic
- Industry benchmarks from TSIA and HDI